Eye on Innovation
machine learning
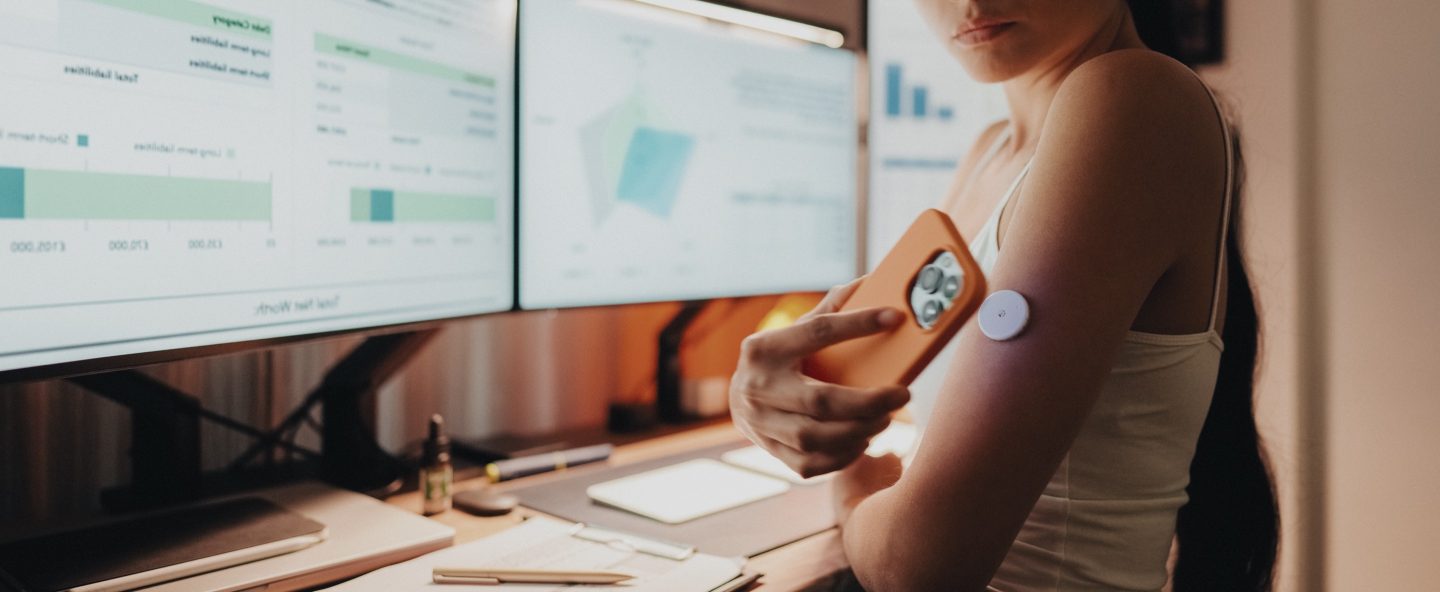
- acceptability (76)
- access to care (169)
- accessibility (74)
- accountability (8)
- accountable care organizations (1)
- accreditation (1)
- accuracy (16)
- adaptation (1)
- ADD/ADHD (1)
- addiction (306)
- adherence (47)
- adolescents (133)
- adoption (23)
- adults (35)
- advertising (12)
- affordability (3)
- alcohol (135)
- algorithm (37)
- Alzheimer’s disease (2)
- American Indian (1)
- anxiety (116)
- Apple (14)
- Apps (1)
- artificial intelligence (181)
- assessment (40)
- audio recording (2)
- autism spectrum disorder (1)
- Avatars (3)
- barriers (8)
- behavior (3)
- behavior change (127)
- behavioral activation (2)
- behavioral health (196)
- bias (10)
- big data (46)
- biomarkers (73)
- biosensor (5)
- Bipolar Disorder (7)
- Black (1)
- blended treatment (5)
- blockchain (1)
- breast cancer (1)
- bullying (1)
- buprenorphine (2)
- burnout (3)
- campaign (1)
- cancer (12)
- cannabis (58)
- care coordination (16)
- Care Management (1)
- caregiver (5)
- CBD (3)
- CBT (54)
- cceptability (1)
- characteristics of inner setting (2)
- characteristics of intervention (3)
- chatbot (12)
- ChatGPT (3)
- child welfare (1)
- children (61)
- chronic disease (75)
- chronic pain (6)
- clinical trials (26)
- clinically validated (1)
- Clinician-Patient Relationship (5)
- clubhouse (1)
- co-occurring disorders (21)
- coaching (1)
- Cocaine (3)
- cognitive behavioral therapy (2)
- cognitive impairment (24)
- collaboration (11)
- collaborative care (2)
- communication (60)
- community (4)
- computer-based (56)
- consumers (35)
- contingency management (15)
- conversational agent (1)
- cost-effectiveness (50)
- county health departments (1)
- COVID-19 (156)
- CTBH (289)
- CTN (14)
- cultural adaptation (2)
- Cybersecurity (15)
- Data (5)
- data analysis (160)
- data collection (142)
- data sharing (31)
- DEA (7)
- decision support (14)
- deep learning (1)
- dementia (3)
- depression (192)
- developers (10)
- development (86)
- diabetes (40)
- diagnosis (61)
- diet (11)
- digital divide (13)
- digital health (472)
- Digital Health Ethics (8)
- Digital Phenotyping (7)
- digital the (1)
- digital therapeutics (76)
- Direct-to-consumer (1)
- disasters (1)
- dissemination (16)
- dissemination and implementation (45)
- diversity (3)
- doctor patient relationship (38)
- e-cigarettes (45)
- ecological momentary assessment (12)
- education (60)
- effectiveness (6)
- efficacy (35)
- electronic health record (59)
- Emergency care (37)
- emergency department (33)
- emotion (19)
- employee assistance program (1)
- engagement (89)
- Epidemiology (3)
- epilepsy (1)
- ethics (21)
- evaluation (23)
- evidence based (33)
- exercise (7)
- Experience Sampling Method (1)
- exposure therapy (1)
- family (1)
- FDA (130)
- feasibility (40)
- fentanyl (12)
- fitness (29)
- funding (10)
- games and gamification (57)
- global health (41)
- global pandemic (3)
- GPS (13)
- hardware (6)
- health access (2)
- health care (69)
- health care delivery (67)
- health care providers (96)
- health care trainees (2)
- health data (135)
- health disparities (31)
- Health Equity (20)
- health information-seeking (5)
- health insurance (2)
- health literacy (12)
- health tracking (5)
- heart rate variability (1)
- help-seeking (1)
- Heroin (2)
- high-risk (30)
- HIPAA (9)
- HIV (41)
- home monitoring (1)
- homelessness (1)
- illicit drugs (85)
- imaging (1)
- implantable device (23)
- implementation (37)
- implementation outcomes (4)
- Implementation Science (2)
- implementation speed (1)
- incentives (18)
- Individual characteristics (1)
- industry (2)
- information visualization (14)
- innovation (1)
- Institutional Review Boards (1)
- integrated care (15)
- internet of medical things (1)
- internet-based (189)
- interoperability (1)
- intervention (222)
- intervention characteristics (4)
- Intervention Development (1)
- IoT (2)
- justice involvement (1)
- juvenile justice (1)
- Latin America (8)
- LGBTQ (2)
- linkage to care (1)
- literature review (58)
- loneliness (3)
- low-income (6)
- machine learning (98)
- maltreatment (1)
- measurement (16)
- measurement-based care (3)
- mechanisms of action (2)
- media (1)
- medical device (6)
- medication management (68)
- Meditation (2)
- memory (1)
- mental health (494)
- mental health care providers (11)
- Mental Illness (14)
- mentorship (1)
- methodology (36)
- mhealth (395)
- mindfulness (19)
- minority groups (5)
- mobile app rating (2)
- mobile apps (463)
- mobile technology (34)
- mobile tools (66)
- mobile-based interventions (3)
- motivation (14)
- motivational interviewing (4)
- movement (1)
- mutual support (1)
- naloxone (2)
- narcan (1)
- Native American (1)
- Natural Language Processing (7)
- neuromodulation (38)
- nicotine (19)
- NIH (50)
- nutrition (1)
- obesity (18)
- observational study (1)
- older adults (12)
- opioid epidemic (209)
- opioid overdose (5)
- opioid use disorder (15)
- opioids (211)
- overdose (108)
- pain (4)
- pain management (86)
- parenting (2)
- Parkinson disease (1)
- participatory design (8)
- patient outcome (11)
- patient visit (1)
- peer support (1)
- personalization (3)
- physica (1)
- physical health (225)
- pilot (1)
- policy and regulation (256)
- post traumatic stress (31)
- precision medicine (20)
- prediction (13)
- pregnancy (26)
- prescription drugs (144)
- prevention (191)
- primary care (25)
- privacy (99)
- problematic technology use (2)
- Project ECHO (1)
- Protection of Human Subjects (1)
- provider attitudes (1)
- psychoeducation (2)
- psychological symptoms (1)
- psychophysiology (1)
- psychosis (1)
- psychosocial (5)
- Psychotic Disorder (3)
- public access (12)
- public health (61)
- qualitative (10)
- quality (8)
- Quality Assurance (1)
- quality improvement (1)
- quality of life (14)
- racial disparities (3)
- radiation therapy (1)
- randomized controlled trial (4)
- recovery (78)
- recovery support (75)
- recruitment (3)
- referral to treatment (10)
- reimbursement (2)
- relapse (34)
- remote monitoring (74)
- researchers (15)
- Retention (4)
- Risk Assessment (2)
- risk behavior (16)
- robotics (2)
- rural (61)
- Safe Station (5)
- safety (96)
- SBIRT (2)
- schizophrenia (4)
- Scoping Review (1)
- Screening (19)
- screening and brief intervention (67)
- security (79)
- self regulation (6)
- self-management (1)
- seniors (29)
- sensors (97)
- serious mental illness (2)
- severe mental illness (60)
- sexual health (2)
- sexually transmitted illnesses (1)
- shared decision making (3)
- sleep (26)
- smartphones (167)
- smoking cessation (62)
- Social Determinants (7)
- social media (119)
- social support (36)
- software (24)
- speech (1)
- stigma (6)
- stimulants (1)
- streaming media (1)
- stress (63)
- students (43)
- substance use (326)
- substance use disorders (98)
- suicide (49)
- survey (5)
- Sustainability (3)
- systematic review (3)
- Technology (17)
- technology-based training (2)
- telehealth (183)
- television (1)
- text messaging (54)
- THC (5)
- theoretical (13)
- therapeutic alliance (1)
- tobacco (100)
- training (9)
- Translation (2)
- trauma (1)
- treatment (414)
- triage tool (1)
- Trust (6)
- undergraduate (1)
- underserved population (3)
- usability (1)
- user characteristics (2)
- user experience (58)
- user-centered design (14)
- validity (3)
- vaping (18)
- veterans (27)
- violence (2)
- virtual reality (68)
- vulnerable populations (88)
- wearable device (201)
- web-based (4)
- weight (1)
- wellness (7)
- withdrawal (20)
- women (31)
- young adults (71)
- Evaluation of an artificial intelligence-based medical device for diagnosis of autism spectrum disorder 07/25/2022
- Development and multimodal validation of a substance misuse algorithm for referral to treatment using artificial intelligence (SMART-AI): A retrospective deep learning study 07/11/2022
- Uncovering heterogeneous associations of disaster-related traumatic experiences with subsequent mental health problems: A machine learning approach 05/23/2022
- How Wearables and Health Apps Can Help Diagnose and Treat Diseases 05/10/2022
- Wearable Technology Promises to Revolutionise Health Care 05/05/2022
- AI Must Be Developed Responsibly to Improve Mental Health Outcomes 05/04/2022
- Designing a Clinical Decision Support Tool That Leverages Machine Learning for Suicide Risk Prediction: Development Study in Partnership With Native American Care Providers 04/11/2022
- New MIT Technique Aims to Boost Fairness Within Machine-Learning Models 03/03/2022
- New Predictive Computer Program Could Help Detect Individuals at High Risk of Depression 01/23/2022